ISI Journal Paper -
Innovative Machine Learning Approaches to Predict the Compressive Strength of Recycled Plastic Aggregate Self-Compacting Concrete Incorporating Different Waste Ashes
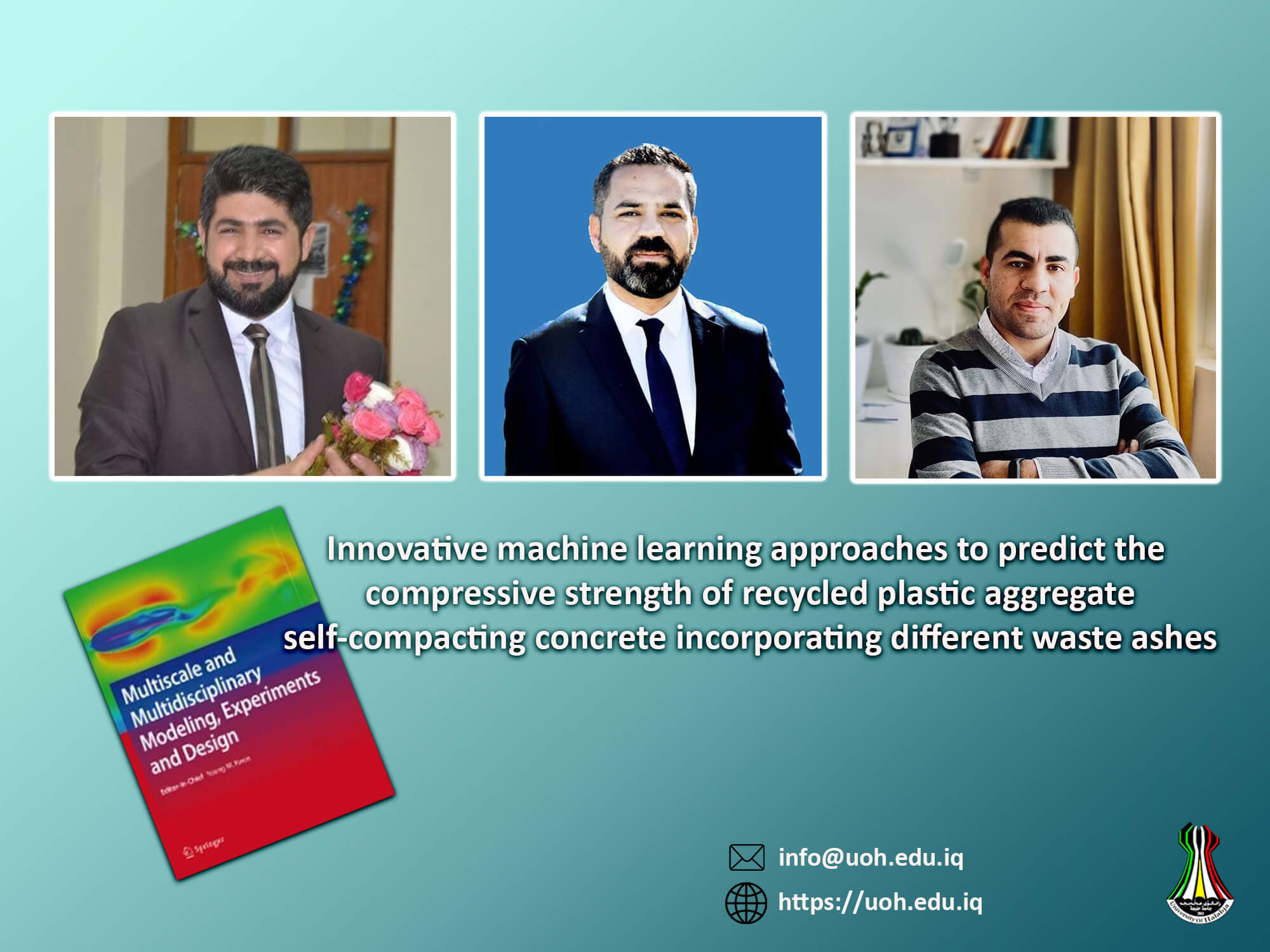
ISI Journal Paper -
Innovative Machine Learning Approaches to Predict the Compressive Strength of Recycled Plastic Aggregate Self-Compacting Concrete Incorporating Different Waste Ashes
Rapid urbanization and industrialization have spurred an increase in concrete manufacturing, contributing to resource depletion and environmental damage. However, this challenge can be addressed by producing concrete from recycled aggregates and industrial waste ashes instead of natural aggregates and cement. Through the blending of diverse by-product ashes and recycled plastic (RP) aggregates, sustainable self-consolidating concrete (SCC) can be created, ensuring the long-term viability of this building material. The development of reliable models to forecast the compressive strength (
��′
) of SCC is crucial for saving money, time, and energy. This research provides valuable insights for scheduling construction projects and determining the optimal time for formwork removal. In this study, four alternative models—Gradient Boosting Regressor (GBR), Extra Tree Regressor (ETR), Deep Neural Network (DNN), and One-Dimensional CNN (1D-CNN)—are suggested for estimating the
��′
of SCC blends composed of RP aggregates. The models were created using a comprehensive dataset containing 400 mixtures, considering various proportions of ten different components and varying curing periods as input factors. Evaluation of the proposed models involved statistical measurements such as Root Mean Squared Error (RMSE), Mean Absolute Error (MAE), Scatter Index (SI), Objective (OBJ) value, and coefficient of determination (R2). The study reveals that the 1D-CNN model emerged as the most efficient among the models for forecasting the
��′
of SCC blends made with RP aggregates, exhibiting RMSE, MAE, OBJ, and R2 values of 2.49 MPa, 1.57 MPa, 1.72 MPa, and 0.99, respectively.